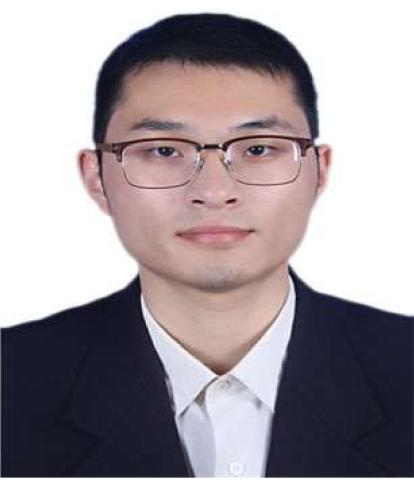
Machine learning-based 3D scan coverage estimation for smart control applications
Date : 13 Janvier 2025
Heure : 14:00
Lieu : M001
Disponible également en visio :
Rejoignez la réunion maintenant
ID de réunion : 386 886 830 171
Code secret : gv7kh3WC
Membre du jury :
NOM et Prénom | Fonction et établissement | Examinateur / Rapporteur / Invité |
M. Nabil ANWE | Professeur, Université Paris-Saclay | Examinateur |
M. Romain RAFFIN | Professeur, Université de Bourgogne | Rapporteur |
M. Mathieu RITOU | Professeur, Ecole Centrale Nantes | Rapporteur |
M. Alexandre DURUPT | Maître de conférences HDR | Examinateur |
Mme. Manon PEUZIN JUBERT | Docteur, société I-MC | Examinateur |
M. Jean-Philippe PERNOT | Professeur, ENSAM | Examinateur |
M. Arnaud POLETTE | Maître de conférences, ENSAM | Examinateur |
M. Ruding LOU | Maître de conférences, ENSAM | Examinateur |
M. Dominique NOZAIS | société I-MC | Invité |
Résumé :
Cette thèse vise à proposer une méthodologie pour prédire a priori la qualité des scans pour des configurations données, afin d'optimiser la planification des points de vue. Elle a d'abord exploré les métriques de qualité et a trouvé que certaines métriques, en particulier la couverture, qui indique la zone de la surface qui serait acquise lors d'une acquisition physique, peuvent être utilisées pour optimiser les configurations de scan. Ensuite, une nouvelle approche basée sur l'apprentissage automatique, 3DSCP-Net, a été proposée pour prédire la couverture a priori. Un nouveau pipeline utilisant la prédiction de la couverture a été proposé pour générer des nuages de points tels que scannés, ce qui illustre que le 3DSCP-Net proposé a une large capacité d'application. Enfin, une résolution du View Planning Problem utilisant la prédiction de couverture et le contrôle du chevauchement a été formulée pour résoudre l'objectif de la thèse, à savoir l'identification de l'ensemble optimal de points de vue afin d'obtenir des acquisitions de haute qualité. Ce travail fournit non seulement un cadre robuste pour l'estimation de la qualité dans la numérisation 3D, mais explore également les applications de l'outil d'estimation de la qualité. Cette recherche contribue à l'objectif plus large d'intégrer l'intelligence artificielle dans l'optimisation des flux de travail d'acquisition 3D, en soutenant les avancées de l'industrie 4.0 et des technologies de jumeaux numériques.
Mots-clés :Lumière structurée, Scan, Mesures de la qualité, Couverture, Apprentissage automatique, Simulation, Jumeau numérique, VPP
Thèse dirigée par : Pr. Jean-Philippe PERNOT, Dr. Arnaud POLETTE, Dr. Ruding LOU
English version :
Machine learning-based 3D scan coverage estimation for smart control applications
Date : 13 Janvier 2025
Time : 2 pm
Location : M001
Also on Teams :
Rejoignez la réunion maintenant
ID de réunion : 386 886 830 171
Code secret : gv7kh3WC
Jury Members :
NAME and First Name | Position and Institution | Reviewer / Rapporteur / Guest |
M. Nabil ANWE | Professeur, Université Paris-Saclay | Reviewer |
M. Romain RAFFIN | Professeur, Université de Bourgogne | Rapporteur |
M. Mathieu RITOU | Professeur, Ecole Centrale Nantes | Rapporteur |
M. Alexandre DURUPT | Maître de conférences HDR | Reviewer |
Mme. Manon PEUZIN JUBERT | Docteur, société I-MC | Reviewer |
M. Jean-Philippe PERNOT | Professeur, ENSAM | Reviewer |
M. Arnaud POLETTE | Maître de conférences, ENSAM | Reviewer |
M. Ruding LOU | Maître de conférences, ENSAM | Reviewer |
M. Dominique NOZAIS | société I-MC | Guest |
Abstract :
This thesis aims to propose a methodology to predict the a priori quality of scans to be obtained from given configurations in order to optimize the resolution of the view planning problem planning. This thesis has first explored the behaviors of the metrics that evaluate the quality of a scan, and found that some metrics, specifically the coverage, which indicates the area of the surface that would be acquired within a specific scan acquisition, can be used to optimize the scan configurations themselves. Then, a novel machine learning-based approach, 3DSCP-Net, has been proposed to predict the a priori coverage. Following, a new pipeline using the coverage prediction has been proposed to generate as-scan point clouds, which illustrates that the proposed 3DSCP-Net has a wide capability of application. The resolution of the View Planning Problem using coverage prediction and with overlapping control has been formulated to solve the objective of the thesis, identifying the optimal set of viewpoints so as to obtain high-quality acquisitions. Experiments have validated that the proposed methodologies can achieve the objectives. This work not only provides a robust framework for quality estimation in 3D scanning but also explores the applications of the quality estimation tool. This research contributes to the broader goal of integrating artificial intelligence into the optimization of 3D acquisition workflows, supporting advancements in Industry 4.0 and digital twin technologies.
Keywords :
Structured light, Scan, Quality metrics, Coverage, Machine learning, Simulation, Digital twin, VPP
Thesis supervised by : Pr. Jean-Philippe PERNOT, Dr. Arnaud POLETTE, Dr. Ruding LOU